
The escalating demand for wireless data traffic, driven by the proliferation of internet-of-things devices and broadband multimedia applications, has intensified the search for innovative solutions in wireless communication.
An advance has been reported in the application of reconfigurable intelligent surfaces for terahertz communications. In a research article published in Intelligent Computing, a team of researchers led by Zhen Gao of Beijing Institute of Technology has introduced a novel physical signal processing method that leverages deep learning to enhance the capabilities of reconfigurable intelligent surface in terahertz communication systems.
Reconfigurable intelligent surface is an innovative technology that passively reflects electromagnetic signals in desired directions by adjusting the phase and amplitude of its elements. This ability to dynamically manipulate signals offers significant advantages over traditional communication systems, especially in indoor environments where the complexity of signal propagation can limit performance.
This technology can be integrated into current terahertz massive multiple-input multiple-output communication systems to passively reflect electromagnetic signals toward desired direction by manipulating phase and amplitude, thereby offering considerable beam forming gain and addressing the inherent challenges of free-space losses and atmospheric attenuation in the terahertz band.
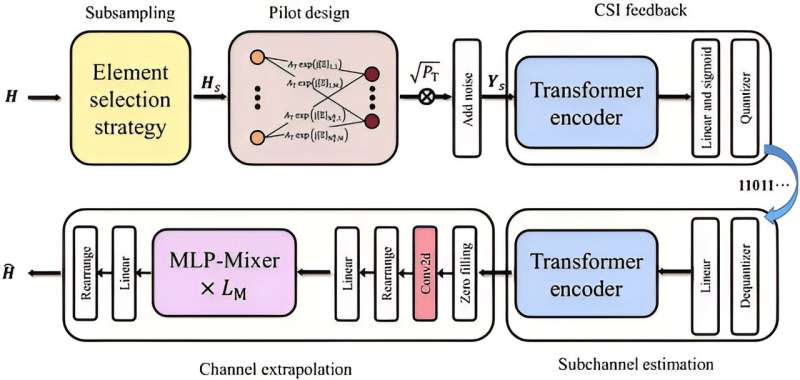
Acquiring accurate channel state information is critical for communication systems that use reconfigurable intelligent surfaces. While solutions based on compressive sensing and deep learning have been explored, challenges persist in terms of computational complexity and storage requirements. Furthermore, existing studies often assume perfect channel state information, overlooking the practical considerations of imperfect channel state information conditions.
The new transmission architecture, based on deep learning, is designed for massive multiple-input, multiple-output terahertz communication systems that use reconfigurable intelligent surfaces. Their channel extrapolation method performs channel state information reconstruction better than conventional alternatives while significantly reducing pilot overhead. Moreover, their beamforming method is more robust to imperfect channel state information.
The research introduces two methods:
- The SFDCExtra method, a spatial-frequency domain channel extrapolation network method that uses deep learning to extrapolate the complete spatial-frequency channel from limited received pilot signals in communication systems that use reconfigurable intelligent surfaces.
- The HBFRPD method, which uses deep learning to design the hybrid beamformer and the refraction phase of the reconfigurable intelligent surface and addresses challenges posed by imperfect channel state information and complex channel characteristics, particularly in indoor scenarios with rich scattering.
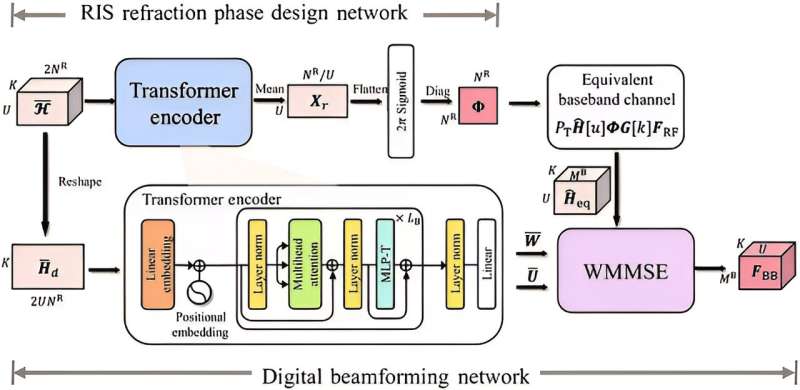
The effectiveness of the methods was evaluated through numerical simulations. The SFDCExtra method aims to enhance the efficiency and accuracy of channel estimation in wireless communication systems. By exploiting spatial-frequency correlations, this method offers promising advancements in channel estimation performance while minimizing pilot overhead.
The researchers conducted a comprehensive evaluation, comparing it against various benchmark algorithms and assessing its robustness under different channel conditions and pilot configurations. Through detailed analyses and performance comparisons, the method showcases effectiveness and versatility in revolutionizing channel estimation methodologies for next-generation communication architectures.
The researchers compared the performance of HBFRPD with other methods in a multi-user communication system. When testing the sum rates achieved by various methods assuming perfect channel state information, they observed that the method outperforms other methods, especially at higher transmit powers, and offers faster computation due to its noniterative nature. Additionally, with imperfect channel state information, inter-user interference affects the sum rates adversely.
The results indicate that HBFRPD remains robust against channel state information errors, outperforming other algorithms in such scenarios. Cumulative distribution functions further support the superior performance, indicating higher probabilities of achieving desired sum rates compared to conventional methods under imperfect channel state information conditions.
More information:
Yang Wang et al, Deep Learning–Based Channel Extrapolation and Multiuser Beamforming for RIS-aided Terahertz Massive MIMO Systems over Hybrid-Field Channels, Intelligent Computing (2023). DOI: 10.34133/icomputing.0065
Intelligent Computing
Deep learning empowers reconfigurable intelligent surfaces in terahertz communication (2024, May 9)
retrieved 9 May 2024
from https://techxplore.com/news/2024-05-deep-empowers-reconfigurable-intelligent-surfaces.html
part may be reproduced without the written permission. The content is provided for information purposes only.

The escalating demand for wireless data traffic, driven by the proliferation of internet-of-things devices and broadband multimedia applications, has intensified the search for innovative solutions in wireless communication.
An advance has been reported in the application of reconfigurable intelligent surfaces for terahertz communications. In a research article published in Intelligent Computing, a team of researchers led by Zhen Gao of Beijing Institute of Technology has introduced a novel physical signal processing method that leverages deep learning to enhance the capabilities of reconfigurable intelligent surface in terahertz communication systems.
Reconfigurable intelligent surface is an innovative technology that passively reflects electromagnetic signals in desired directions by adjusting the phase and amplitude of its elements. This ability to dynamically manipulate signals offers significant advantages over traditional communication systems, especially in indoor environments where the complexity of signal propagation can limit performance.
This technology can be integrated into current terahertz massive multiple-input multiple-output communication systems to passively reflect electromagnetic signals toward desired direction by manipulating phase and amplitude, thereby offering considerable beam forming gain and addressing the inherent challenges of free-space losses and atmospheric attenuation in the terahertz band.
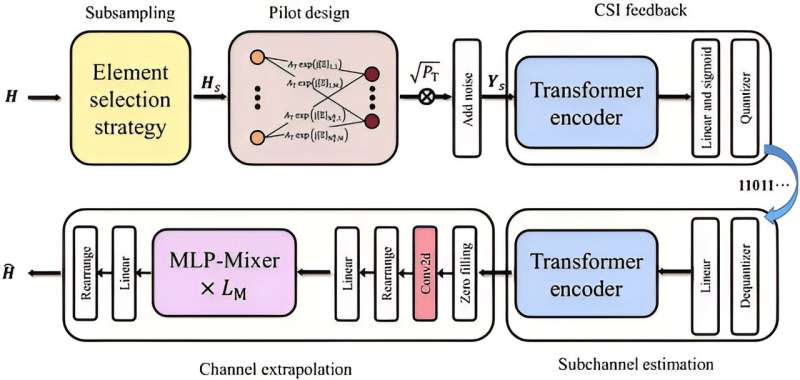
Acquiring accurate channel state information is critical for communication systems that use reconfigurable intelligent surfaces. While solutions based on compressive sensing and deep learning have been explored, challenges persist in terms of computational complexity and storage requirements. Furthermore, existing studies often assume perfect channel state information, overlooking the practical considerations of imperfect channel state information conditions.
The new transmission architecture, based on deep learning, is designed for massive multiple-input, multiple-output terahertz communication systems that use reconfigurable intelligent surfaces. Their channel extrapolation method performs channel state information reconstruction better than conventional alternatives while significantly reducing pilot overhead. Moreover, their beamforming method is more robust to imperfect channel state information.
The research introduces two methods:
- The SFDCExtra method, a spatial-frequency domain channel extrapolation network method that uses deep learning to extrapolate the complete spatial-frequency channel from limited received pilot signals in communication systems that use reconfigurable intelligent surfaces.
- The HBFRPD method, which uses deep learning to design the hybrid beamformer and the refraction phase of the reconfigurable intelligent surface and addresses challenges posed by imperfect channel state information and complex channel characteristics, particularly in indoor scenarios with rich scattering.
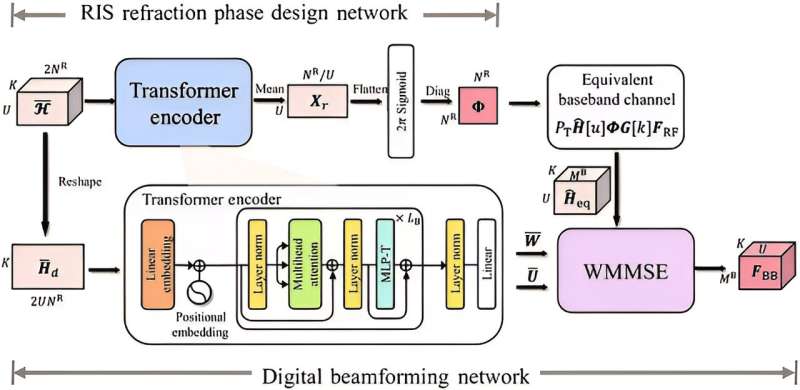
The effectiveness of the methods was evaluated through numerical simulations. The SFDCExtra method aims to enhance the efficiency and accuracy of channel estimation in wireless communication systems. By exploiting spatial-frequency correlations, this method offers promising advancements in channel estimation performance while minimizing pilot overhead.
The researchers conducted a comprehensive evaluation, comparing it against various benchmark algorithms and assessing its robustness under different channel conditions and pilot configurations. Through detailed analyses and performance comparisons, the method showcases effectiveness and versatility in revolutionizing channel estimation methodologies for next-generation communication architectures.
The researchers compared the performance of HBFRPD with other methods in a multi-user communication system. When testing the sum rates achieved by various methods assuming perfect channel state information, they observed that the method outperforms other methods, especially at higher transmit powers, and offers faster computation due to its noniterative nature. Additionally, with imperfect channel state information, inter-user interference affects the sum rates adversely.
The results indicate that HBFRPD remains robust against channel state information errors, outperforming other algorithms in such scenarios. Cumulative distribution functions further support the superior performance, indicating higher probabilities of achieving desired sum rates compared to conventional methods under imperfect channel state information conditions.
More information:
Yang Wang et al, Deep Learning–Based Channel Extrapolation and Multiuser Beamforming for RIS-aided Terahertz Massive MIMO Systems over Hybrid-Field Channels, Intelligent Computing (2023). DOI: 10.34133/icomputing.0065
Intelligent Computing
Deep learning empowers reconfigurable intelligent surfaces in terahertz communication (2024, May 9)
retrieved 9 May 2024
from https://techxplore.com/news/2024-05-deep-empowers-reconfigurable-intelligent-surfaces.html
part may be reproduced without the written permission. The content is provided for information purposes only.